Multi-label Classification with High-rank and High-order Label Correlations
Sep 6, 2023·
,,,,,·
0 min read

Chongjie Si
Yuheng Jia
Ran Wang
Min-Ling Zhang
Yanghe Feng
Chongxiao Qu
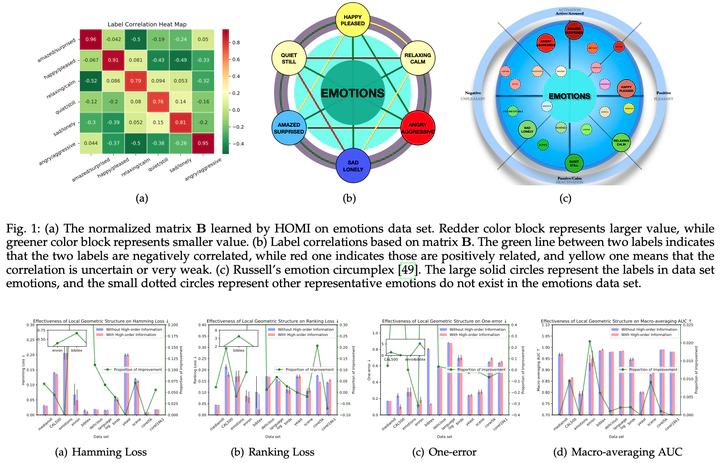
Abstract
Exploiting label correlations is important to multi-label classification. Previous methods capture the high-order label correlations mainly by transforming the label matrix to a latent label space with low-rank matrix factorization. However, the label matrix is generally a full-rank or approximate full-rank matrix, making the low-rank factorization inappropriate. Besides, in the latent space, the label correlations will become implicit. To this end, we propose a simple yet effective method to depict the high-order label correlations explicitly, and at the same time maintain the high-rank of the label matrix. Moreover, we estimate the label correlations and infer model parameters simultaneously via the local geometric structure of the input to achieve mutual enhancement. Comparative studies over twelve benchmark data sets validate the effectiveness of the proposed algorithm in multi-label classification. The exploited high-order label correlations are consistent with common sense empirically.
Publication
IEEE Transactions on Knowledge and Data Engineering