Unleashing the Power of Task-Specific Directions in Parameter Efficient Fine-tuning
Jan 23, 2025·
,,,,,·
0 min read

Chongjie Si
Zhiyi Shi
Shifan Zhang
Xiaokang Yang
Hanspeter Pfister
Wei Shen
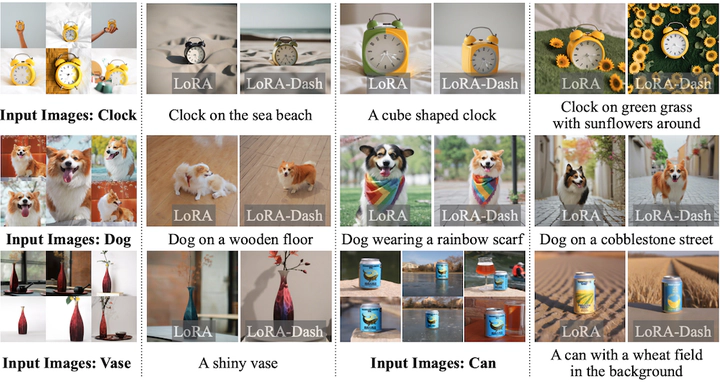
Abstract
Large language models demonstrate impressive performance on downstream tasks, yet requiring extensive resource consumption when fully fine-tuning all parameters. To mitigate this, Parameter Efficient Fine-Tuning (PEFT) strategies, such as LoRA, have been developed. In this paper, we delve into the concept of task-specific directions (TSDs)—critical for transitioning large models from pretrained states to task-specific enhancements in PEFT. We propose a framework to clearly define these directions and explore their properties, and practical utilization challenges. We then introduce a novel approach, LoRA-Dash, which aims to maximize the impact of TSDs during the fine-tuning process, thereby enhancing model performance on targeted tasks. Extensive experiments have conclusively demonstrated the effectiveness of LoRA-Dash, and in-depth analyses further reveal the underlying mechanisms of LoRA-Dash.
Publication
The Thirteenth International Conference on Learning Representations